Selected Projects
Soil Sensing and Nutrient management
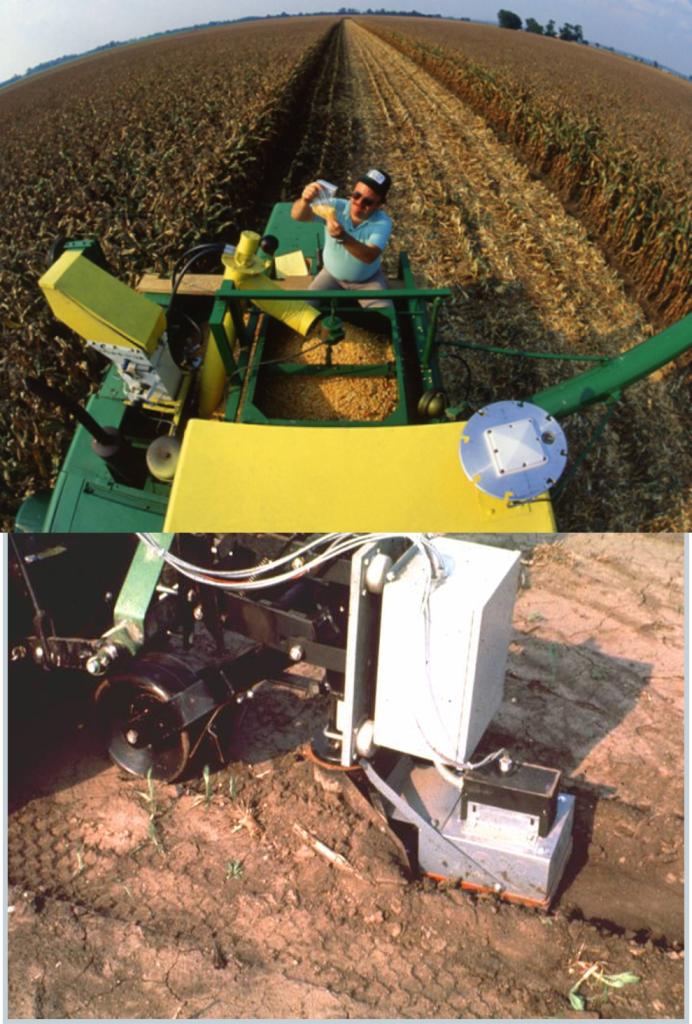
At the heart of our project is a commitment to revolutionizing precision agriculture. We are harnessing the power of advanced sensing technologies and decision support systems to drastically improve both productivity and sustainability in farming.
Our Approach:
- Smart Water Management: Utilizing soil electrical conductivity and moisture data for efficient water usage and better crop health.
- 3D Soil Modeling: Advanced models from dense sensor data offer in-depth soil analysis, aiding optimal crop growth.
- Innovative Smart Firmer Sensor: This tool predicts soil organic matter and measures key parameters like temperature and moisture.
- Spectral Scanning: Assessing various soil properties, including fertility, health, and texture, for comprehensive soil insights.
Complete Farm Digitalization
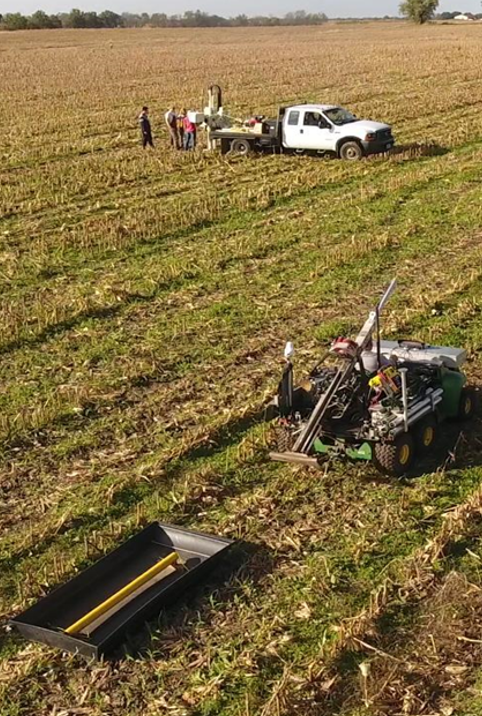
Our initiative is at the forefront of agricultural innovation, focusing on the complete digitization of large-scale farms. We’re integrating cutting-edge technology to create a detailed, accurate digital representation of the agricultural landscape.
Our Approach:
- Comprehensive Data Integration: We combine digital elevation models, soil electrical conductivity (EC), fertility, and health data for a complete soil analysis.
- Advanced Sensing Technologies: Utilizing smart ground-based sensors and state-of-the-art remote sensing suits, we gather extensive, precise data.
- High-Accuracy Topographic Models: Our models are enriched with data from RTK-integrated UAVs and ground surveying systems, offering unparalleled topographic accuracy.
UAV Swarm Management for Soybean Defoliation Monitoring
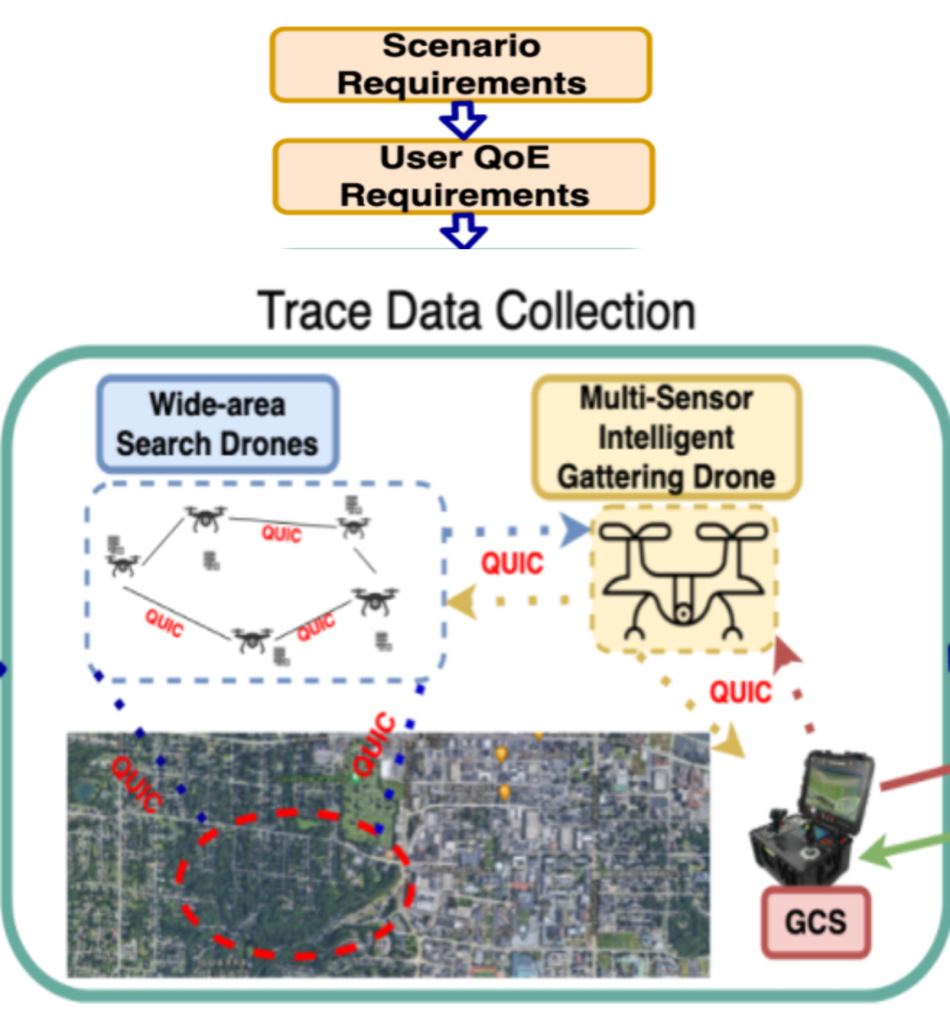
Our team is at the forefront of agricultural innovation, developing intelligent drone swarm technology for efficient and extensive crop scouting. We are redefining how fields are mapped and monitored, bringing precision and efficiency to farming practices.
Project Highlights:
- Long-Distance Field Mapping: Our UAVs can map entire fields from significant distances, offering comprehensive coverage.
- Smart Drone Coordination: We focus on creating a learning-based system for efficient task scheduling among large drone swarms in agricultural applications.
- Focused Soybean Defoliation Study: Utilizing a network of multiple UAVs and a central control system, we are advancing soybean defoliation modeling.
Innovating Ergonomic Tool Design with Digital Technology
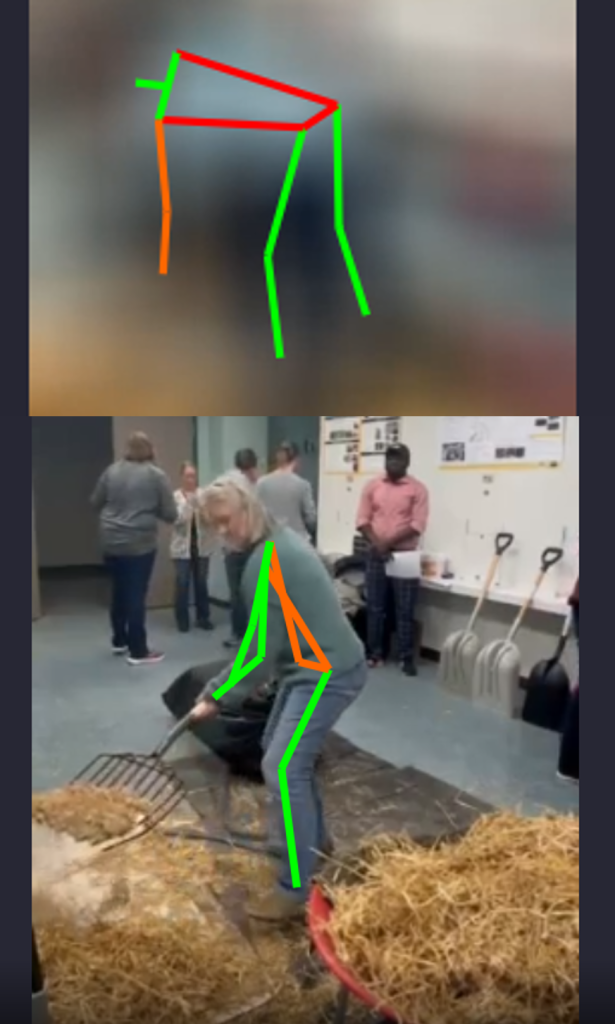
Welcome to our initiative for a safer, healthier farming future for the women who run nearly a third of American farms. We’re closing the gap with specially designed, ergonomic tools and educational initiatives. By harnessing smart technology, we’re tailoring farm equipment to fit women’s needs, backed by thorough research and real-world testing.
Project Highlights:
- Ergonomically Designed Tools: Recognizing the unique physical requirements of women farmers, we are developing ergonomic tools. These are not only adaptations of existing equipment but also new designs that consider factors like size, weight, and ease of use.
- Smart Technology Integration: At the heart of our project is the application of smart technology. We’re leveraging advanced systems, automation, and AI to create equipment that’s more intuitive and responsive to the needs of women in agriculture.