Innovating Drone-Edge-Cloud Orchestration
Project Overview: In the realm of smart agriculture, we are at the forefront of developing a cutting-edge hierarchical learning-based drone-edge cloud orchestration system. This system is designed to intelligently schedule and manage tasks for massive drone swarms, optimizing their use in a variety of agricultural applications.
Key Research Challenges:
- Long-Distance UAV Design for Large Crop Fields: One of our primary challenges is engineering UAVs capable of traversing large distances, often exceeding 100 acres, from their takeoff point. The goal is to map entire fields in an energy-efficient and effective manner, ensuring comprehensive coverage and data collection.
- High-Resolution Imaging for Agricultural Monitoring: A critical requirement in agricultural monitoring is acquiring high ground-sample distance images. We are tackling the challenge of managing UAV flyover heights and mission durations to ensure these high-quality imaging needs are met, aligning with the evolving demands of smart farming.
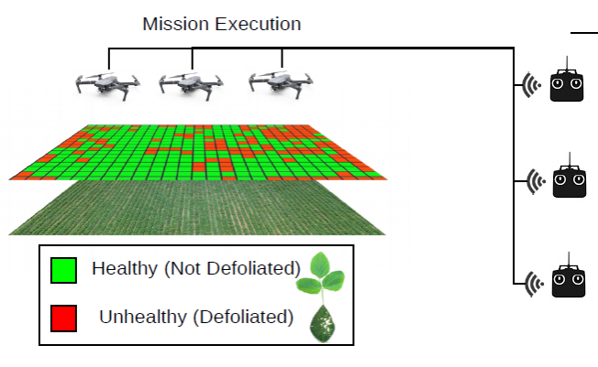
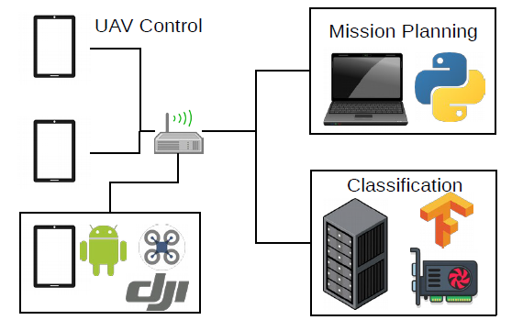
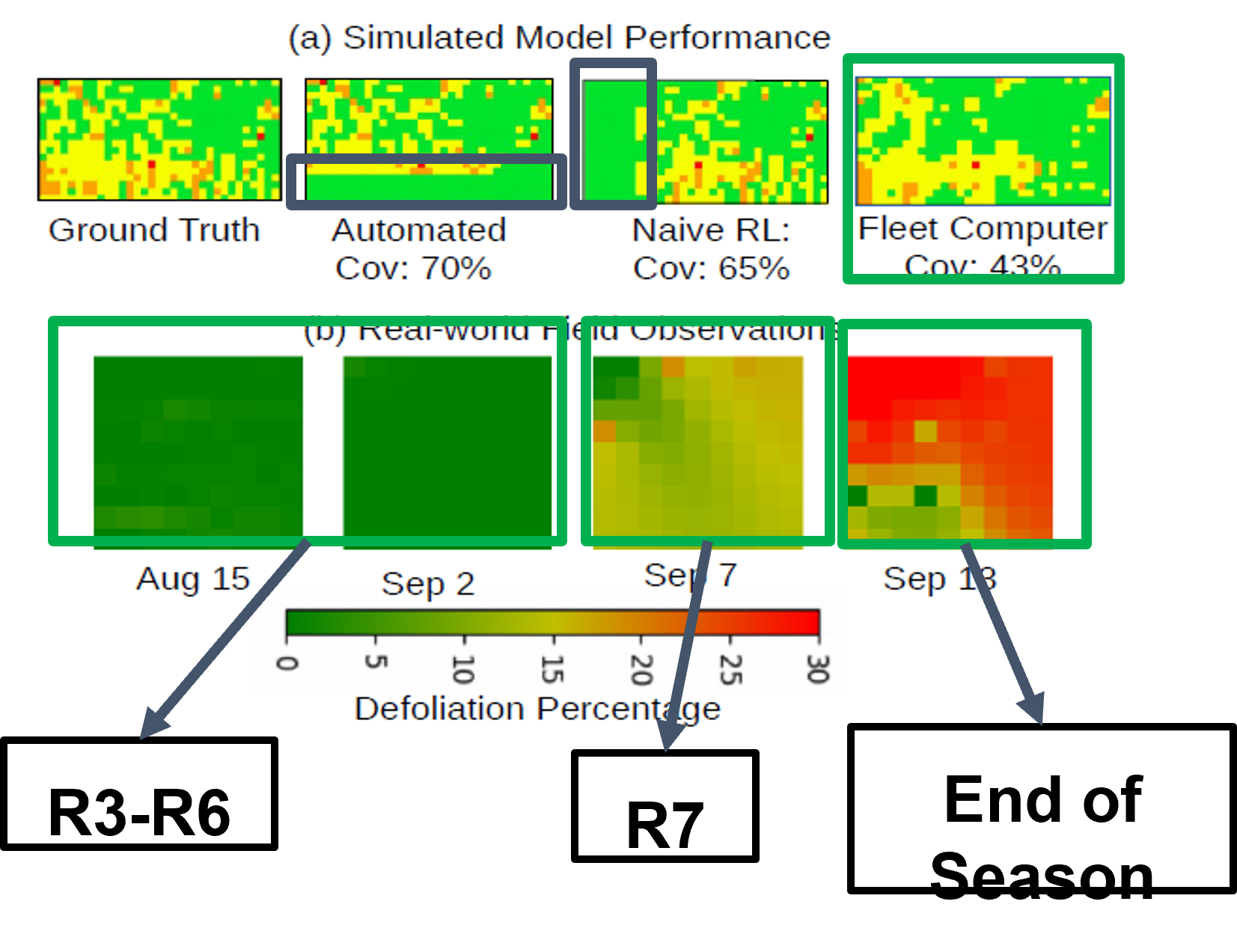
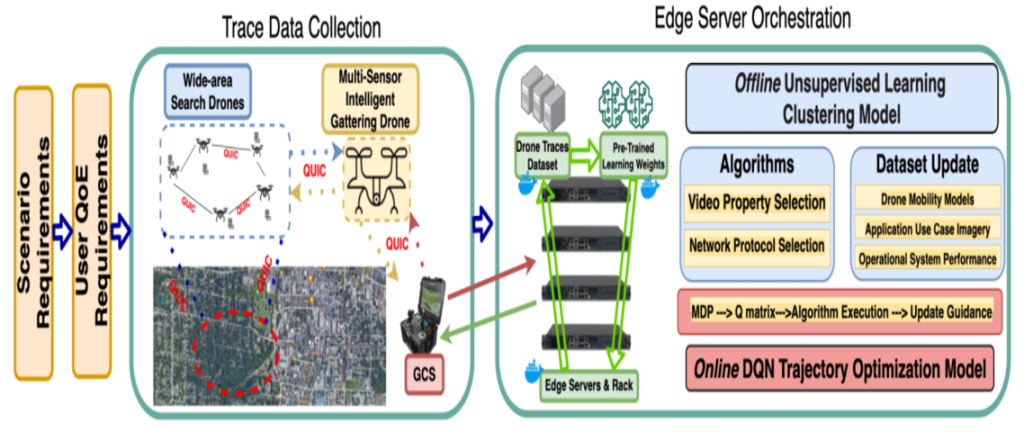
Project Overview: In the realm of smart agriculture, we are at the forefront of developing a cutting-edge hierarchical learning-based drone-edge cloud orchestration system. This system is designed to intelligently schedule and manage tasks for massive drone swarms, optimizing their use in a variety of agricultural applications.
Key Research Challenges:
- Long-Distance UAV Design for Large Crop Fields: One of our primary challenges is engineering UAVs capable of traversing large distances, often exceeding 100 acres, from their takeoff point. The goal is to map entire fields in an energy-efficient and effective manner, ensuring comprehensive coverage and data collection.
- High-Resolution Imaging for Agricultural Monitoring: A critical req
Novel Research Achievements: Our project showcases innovative strides in Intelligent Drone Swarm Crop Scouting within a Soybean Defoliation Modeling Scenario. Utilizing three DJI Mavic UAVs, multiple flight controllers, a central ground control station, and a compute node, we’ve established a more systematic and efficient approach to field sampling.
Efficiency Breakthrough: Our experimental design reveals that a fleet computer model achieves more uniform field sampling. This strategy requires only 43% field coverage to produce an 80% accurate map.
Lifecycle Tracking: We can now monitor the entire growth cycle of soybean crops, capturing the natural defoliation process towards the season’s end, which is vital for crop management and yield prediction.
RL-Based Modeling: Leveraging reinforcement learning, our UAV swarms perform efficiently, accurately, and reproducibly, demonstrating the effectiveness of RL-based models for soybean defoliation analysis.
Reinforcement Learning Performance: Our approach merges reinforcement learning performance with in-field observations to enhance soybean defoliation modeling, offering a glimpse into the future of agricultural technology.
Join Our Agritech Revolution:
Explore our technology that’s setting new standards in precision farming and sustainable agriculture.