Project Overview:
Soil is one of the limiting factors for crop growth, yield, and grain quality. Emerging farming practices, such as cover crops, precision nitrogen management, are developed to rehabilitate soil health. However, there are no credible, verifiable, and cost-effective frameworks to quality cropland soil health at the field level. This study aimed to provide an accurate and scalable solution to quantify soil health using unmanned arial vehicle (UAV)-based remote sensing technology as biosensor for digital soil mapping (DSM). Research experiments were conducted in Bradford soybean fields with different soil fertility, cropping diversity (e.g., cover crops, rotation), and landscape positions. Different types of UAV imagery, including high-resolution RGB, near-infrared (NIR), red-edge and IR thermal images, were collected weekly or bi-weekly from emergence to harvest stage. Imagery data were processed to calculated geo-referenced image features and vegetation indices (VIs). Weather information (i.e., air temperature, precipitation) was recorded using nearby weather stations. Soil moisture was monitored using multiple-depth soil moisture sensors. Soil electrical conductivity (ECa) was captured by Veris 3100. Digital elevation model (DEM) was produced by UAV-based LiDAR imagery. Soil samples were collected at 69 locations in two depths (0 – 2 inches, and 2 – 6 inches) for soil health analysis and fertility assessment. Soybean was harvested using a combine harvester equipped with yield monitor system to generate yield maps. The objectives of this study are: 1) Exploring the geostatistical (Empirical Bayesian Kriging), machine learning (Random Forest and Geographically Weighted Regression), and hybrid (Regression Kriging) DSM methods to predict soil health and fertility using ArcGIS Pro with multi-source sensing data (yield map, digital elevation model, ECa, and VIs). 2) Improving crop growth and soil health simulations by incorporating time-series observation data (VIs, soil moisture, weather information) into process-based model with data assimilation (Ensemble Kalman Filter). 3) Providing new insights for soybean production by multi-objective optimal management zone delineation and driven-factors analysis of DSM. The research results are expected to be adapted by US soybean farmers to continue soybean profitability, sustainability, and climate change resilience.
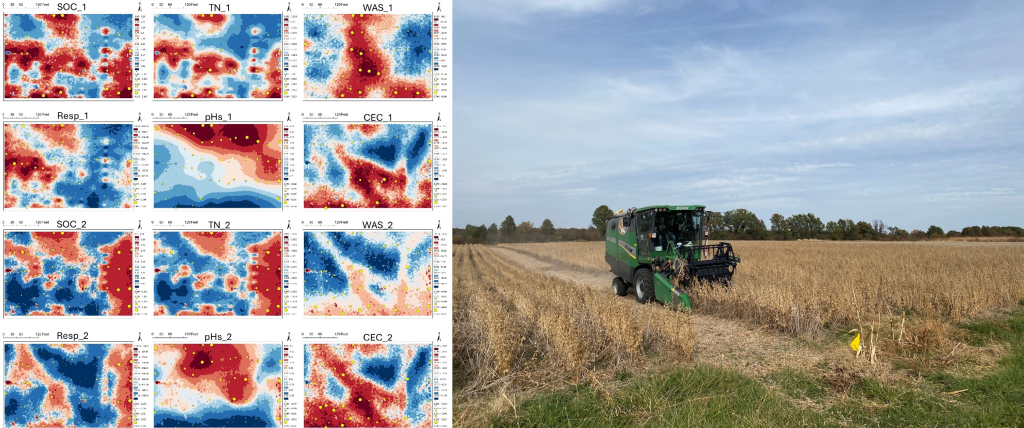