This research explores AI-driven agricultural solutions by integrating machine learning, remote sensing, and automation to enhance crop monitoring, yield estimation, and precision management.
In automated corn yield assessment, a YOLOv11-X + BoT-SORT tracking system achieves 99% accuracy in detecting corn ears during harvest. Future work aims to develop a pipeline for estimating kernel and row counts. Meanwhile, multispectral and hyperspectral imaging play a key role in monitoring crop health, with findings showing that combining multispectral imagery with soil ECa improves chlorophyll predictions. For nitrogen estimation, a newly developed vegetation index (FD588 nm – FD1667 nm / FD588 nm + FD1667 nm – FD1205 nm) shows a strong correlation (r = 0.93) with leaf nitrogen content.
A review on hyperspectral sensing highlights the increasing adoption of UAV-based sensors since 2021, with PLSR emerging as the most reliable model for nitrogen estimation. Key spectral regions in the 518-925 nm range, particularly around 713 nm, show high relevance, though further studies are needed to refine SWIR-based assessments.
In cotton emergence studies, UAV-based imagery reveals that soil ECa and elevation influence plant uniformity (R² = 0.62), while a machine learning model predicts emergence rates with R² = 0.75 based on environmental data. Similarly, for dicamba damage assessment, deep learning proves more effective than traditional methods, with a DenseNet-121 transfer learning model achieving 82% accuracy.
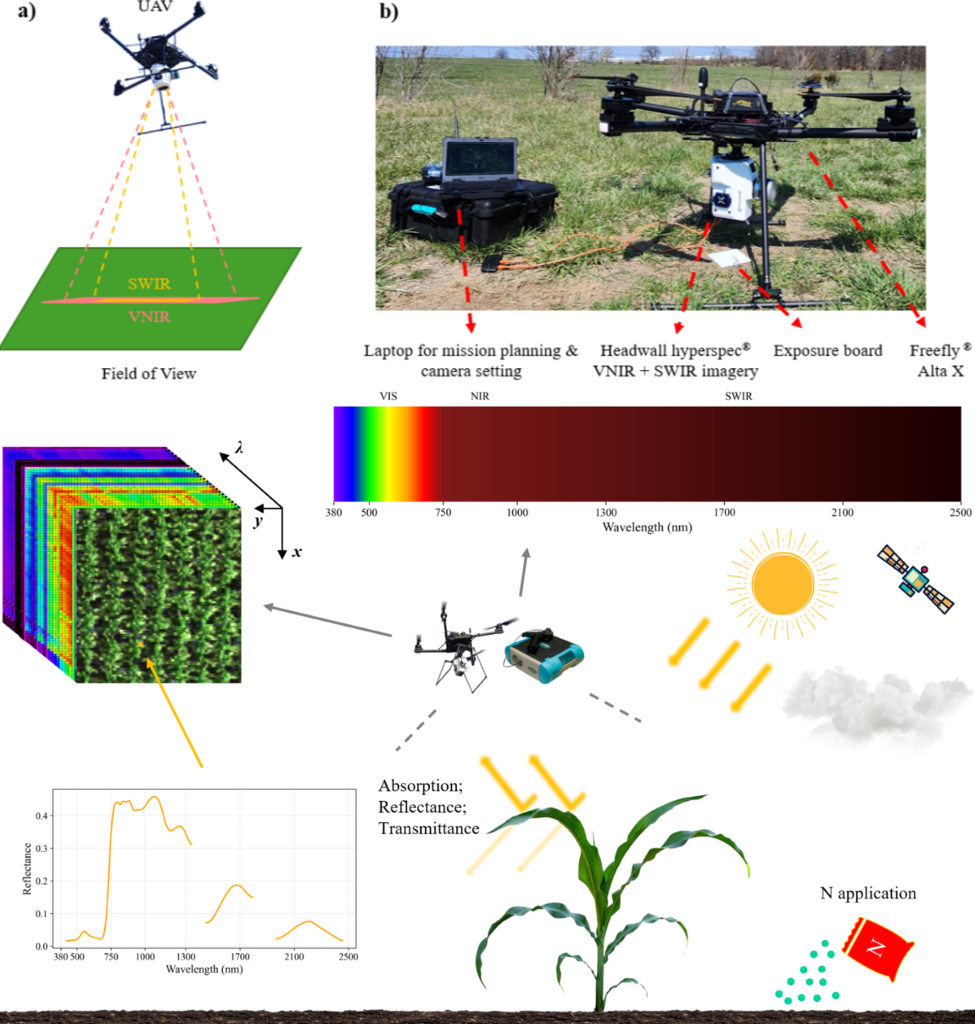
By refining computational pipelines and leveraging remote sensing technologies, this research contributes to the advancement of scalable, AI-driven precision agriculture solutions.